Putting intelligent search to work
The progression of enterprise search is one of the most profound developments of the contemporary data ecosystem.
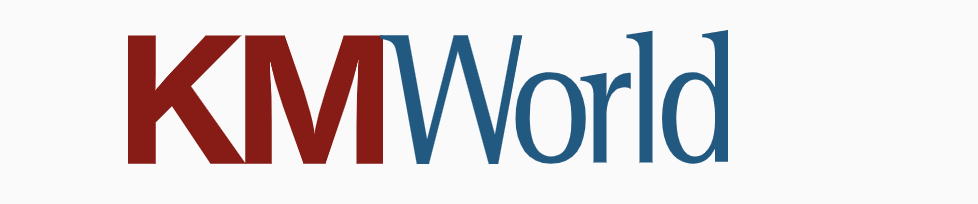
Arria CTO, Neil Burnett, offers his insight in this KMWorld article. Below is an excerpt and a link to the full text.
The progression of enterprise search is one of the most profound developments of the contemporary data ecosystem. While expanding to utilize almost every dimension of cognitive computing, it reinforces some of the most pivotal aspects of knowledge management, business intelligence, and real-time querying.
In the process, search has moved beyond a heavy reliance on mere keywords to encompass something much more: seamless (and seemingly effortless) natural language interactions between enterprise users and their data.
Neil Burnett, CTO at Arria NLG says: "Cognitive search involves analytics—not just indices and basic retrieval—which is integral to natural language generation systems. “The right analytics in your system lets you answer more and more questions,” observed “In some cases, it’s all just purely statistical stuff. Then, there are more advanced approaches—not just for simple outliers but [for identifying] what’s an anomaly in context or over an extended period of time.”
According to Qlik CTO Mike Potter, “The transition from a query to a question is really the evolution of search in terms of your ability to get the most out of the data itself. It’s more than just questions and answers: It’s more about a conversation.”
Conversational engagements with data systems via cognitive search rely on several aspects of AI, including these:
♦ Conversational AI: Formerly known as natural language interaction, conversational AI enables users to ask questions in natural language and get responses the same way.
♦ Symbolic reasoning: This rules-based form of AI’s traditional knowledgebase relies heavily on taxonomies, knowledge graphs, and fundamental understanding of business concepts.
♦ Machine learning: Often employed with deep neural networks, various machine learning approaches—including both supervised and unsupervised learning—are essential for analyzing data to produce rapid search responses.
♦ Neuro-symbolic AI: The fusion of AI’s statistical or machine learning foundation with its knowledge or symbolic reasoning side overcomes the shortfalls of each respective approach to optimize search capabilities.
AI enables search to surmount its basic keyword limitations to become a nuanced form of simplistic linguistic interactions, and the overarching utility provided by search itself has also burgeoned into more than previously offered, even a short while ago.
Computational linguistics: This discipline enables cognitive search systems to “choose the right words to articulate findings properly,” Burnett said. “It describes the patterns that have been detected, but in a way that contextualizes them so the reader will understand and get the meaning without looking through lots and lots of graphs, which may be confusing.”